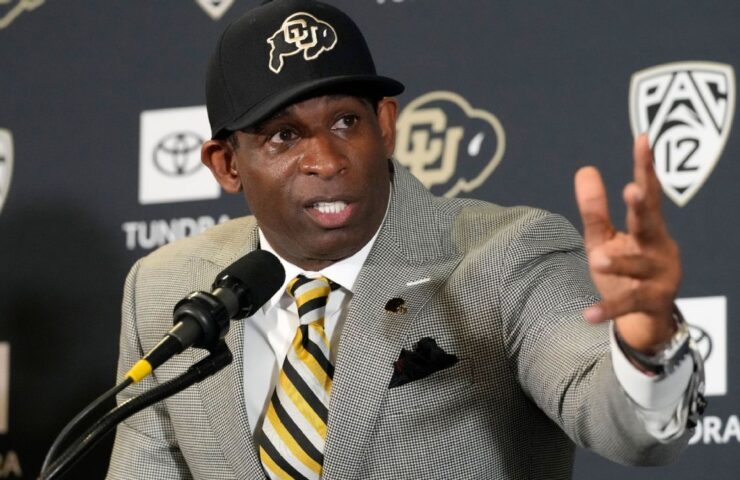
Connelly on transfer patterns: What last season can teach us
The 2022 college football season was plenty of things. It was the year of the growing Georgia dynasty. It was the year of terrific playoff semifinals.Most of all, however, it
was the year of the transfer. Thanks to the NCAA’s long-time-coming decision to allow first-time transfers to change schools without sitting for a year, and thanks to the general proliferation of the transfer website as a method for roster structure, FBS coaches made full usage of short-term talent.A years back, it was a quite big offer if a school took 5 or 6 transfers in one class; in 2022, FBS groups balanced more than 7. It was a dizzying experience.Last summer, I had a look at the trends I found from portal usage as we headed into such a transfer-heavy season. Now that 2022 is over and we’ve turned the page to 2023, let’s take on the very same exercise.Once once again, I am utilizing stats to compare what players produced at their previous schools in 2021 (or, where suitable, previously seasons)to what they produced at their 2022 destinations.Because the requirements of various positions are so different, and due to the fact that the data for measuring efficiency at provided positions are too, I again kept things simple. I developed a quick scoring system based mainly on two things: How much did a transfer play, and how well did he play?Loosely speaking, the scoring scale was set up like this:1. Nearly never played(or dipped into a level lower than FBS) 2. Played a little 3. Played a fair bit and
performed at an average or worse level 4. Played quite a bit and did well 5. Absolute star, among the best in college football at their position
(Note: For this exercise
, I looked only at offending and defensive players, not specialists. Apologies to all the kickers and punters out there.)I offered players a score for their efficiency at both their traditional
and their new school, then took a look at the resulting changes and averages. This is a very subjective procedure, however the objective was to scope out macro-level trend information for a significantly substantial data set, and this was a solid process for achieving that.